Humans Can't Be the Sole Keepers of Scientific Knowledge | WIRED
Published by Reblogs - Credits in Posts,
Humans Can't Be the Sole Keepers of Scientific Knowledge | WIRED
Humans Can't Be the Sole Keepers of Scientific Knowledge
There’s an old joke that physicists like to tell: Everything has already been discovered and reported in a Russian journal in the 1960s, we just don’t know about it. Though hyperbolic, the joke accurately captures the current state of affairs. The volume of knowledge is vast and growing quickly: The number of scientific articles posted on arXiv (the largest and most popular preprint server) in 2021 is expected to reach 190,000—and that’s just a subset of the scientific literature produced this year.
It’s clear that we do not really know what we know, because nobody can read the entire literature even in their own narrow field (which includes, in addition to journal articles, PhD theses, lab notes, slides, white papers, technical notes, and reports). Indeed, it’s entirely possible that in this mountain of papers, answers to many questions lie hidden, important discoveries have been overlooked or forgotten, and connections remain concealed.
Artificial intelligence is one potential solution. Algorithms can already analyze text without human supervision to find relations between words that help uncover knowledge. But far more can be achieved if we move away from writing traditional scientific articles whose style and structure has hardly changed in the past hundred years.
Text mining comes with a number of limitations, including access to the full text of papers and legal concerns. But most importantly, AI does not really understand concepts and the relationships between them, and is sensitive to biases in the data set, like the selection of papers it analyzes. It is hard for AI—and, in fact, even for a nonexpert human reader—to understand scientific papers in part because the use of jargon varies from one discipline to another and the same term might be used with completely different meanings in different fields. The increasing interdisciplinarity of research means that it is often difficult to define a topic precisely using a combination of keywords in order to discover all the relevant papers. Making connections and (re)discovering similar concepts is hard even for the brightest minds.
As long as this is the case, AI cannot be trusted and humans will need to double-check everything an AI outputs after text-mining, a tedious task that defies the very purpose of using AI. To solve this problem we need to make science papers not only machine-readable but machine-understandable, by (re)writing them in a special type of programming language. In other words: Teach science to machines in the language they understand.
Writing scientific knowledge in a programming-like language will be dry, but it will be sustainable, because new concepts will be directly added to the library of science that machines understand. Plus, as machines are taught more scientific facts, they will be able to help scientists streamline their logical arguments; spot errors, inconsistencies, plagiarism, and duplications; and highlight connections. AI with an understanding of physical laws is more powerful than AI trained on data alone, so science-savvy machines will be able to help future discoveries. Machines with a great knowledge of science could assist rather than replace human scientists.
Mathematicians have already started this process of translation. They are teaching mathematics to computers by writing theorems and proofs in languages like Lean. Lean is a proof assistant and programming language in which one can introduce mathematical concepts in the form of objects. Using the known objects, Lean can reason whether a statement is true or false, hence helping mathematicians verify proofs and identify places where their logic is insufficiently rigorous. The more mathematics Lean knows, the more it can do. The Xena Project at Imperial College London is aiming to input the entire undergraduate mathematics curriculum in Lean. One day, proof assistants may help mathematicians do research by checking their reasoning and searching the vast mathematics knowledge they possess.
Writing mathematics in a language like Lean is arguably more straightforward than in other areas of science. Of course, not all scientific results could be rewritten in this way, but many, especially in STEM fields, can be. In designing this new language, one might start from something like Lean and customize it, adding features specific to that field. To be sure, there is more to defining a scientific idea than mathematics; there is context, intuition, and interpretation. This is why, despite quantum mechanics having a very clear mathematical description, there are countless articles and textbooks attempting to explain it. It will be challenging to convey these subtle aspects of scientific ideas to machines, but remember that the very purpose of machine assistants is to help the human scientist refine these deeper points and express them more clearly. Perhaps precisely because some scientific concepts defy human intuition, machines will be better placed to put them in context.
See What’s Next in Tech With the Fast Forward Newsletter
We have yet to develop this common language of humans and machines, which will likely evolve to have field-specific vocabularies. But when we do, there will be no shortage of early adopters. As the Xena Project has shown, the digital native generations can learn new languages very quickly without prior programming experience. For some scientists, this language may even be more straightforward than writing prose in English, which may not be their mother tongue. It would help them better structure ideas. Interpreters can translate Lean back to math, and in a similar way the new language could be interpreted to English or any other language for nonexperts.
Translating most of the existing knowledge for machines is a gigantic undertaking, yet not an impossible one. Scientists are good at creating new ways of sharing information, from the World Wide Web to preprint servers like arXiv. It’s not outlandish to imagine each scientist contributing to the library of scientific concepts translated for machines. As in mathematics, other undergraduate curricula can be taught to machines by students taking the courses. Graduate students would input the scientific concepts relevant to their topic and researchers would directly write their new results in the new language.
This endeavor would take a lot of time and money, in addition to collective effort. But there may be no other way to tackle the ever-growing volume of scientific knowledge: We’ll keep wasting time and resources rediscovering known concepts and pursuing dead-end roads. The future of science can only be a human-machine enterprise.
- The latest on tech, science, and more: Get our newsletters!
- Looks that quill: The dark side of hedgehog Instagram
- Climate change is making it harder to flee disasters
- I'm a Lyft driver. Passengers act like I'm part of the app
- Covid has created a virtual Renaissance for life drawing
- The US AI industry risks becoming winner-take-most
- ️ Explore AI like never before with our new database
- WIRED Games: Get the latest tips, reviews, and more
- Things not sounding right? Check out our favorite wireless headphones, soundbars, and Bluetooth speakers
© 2021 Condé Nast. All rights reserved. Use of this site constitutes acceptance of our User Agreement and Privacy Policy and Cookie Statement and Your California Privacy Rights. Wired may earn a portion of sales from products that are purchased through our site as part of our Affiliate Partnerships with retailers. The material on this site may not be reproduced, distributed, transmitted, cached or otherwise used, except with the prior written permission of Condé Nast. Ad Choices
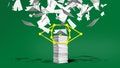
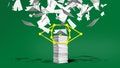
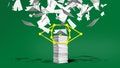
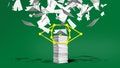
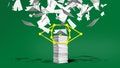
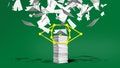
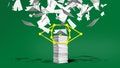
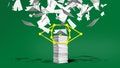
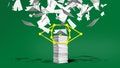
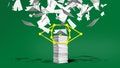
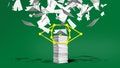
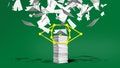